Alpha and Beta risks are the risks involved while conducting a statistical analysis with the help of Hypothesis testing. While estimating a value with Hypothesis testing, it is possible that two types of mistakes occur:
- Incorrectly deciding that the value is out of the predicted range (Rejecting a true hypothesis), and
- Incorrectly deciding that an outside value falls within the predicted range (Accepting a false hypothesis).
These two are respectively called as Alpha and Beta Error or Risk in statistical terms. Let us look at the two concepts in detail.
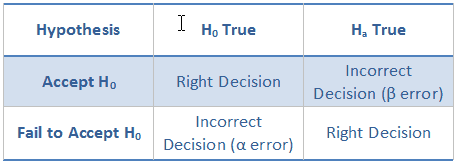
Alpha Error:
Error occurring when a Null hypothesis is rejected, when it is true. This is also called as Type I error, Producer’s risk, Level of Significance etc. To reduce or control this, a value called as p-value is calculated for the hypothesis test. The p-value should be less than or equal to the Significance Level chosen for the test. Therefore by choosing a higher significance value (which is in Percentage), alpha error or risk can be controlled. For example, A producer can test his pump’s diameter to fall between 9.95cm to 10.05 cm. He can choose a significance level of 95% which allows 5% error Thus p-value will be 0.05. To be more accurate if he chooses a significance level of 99%, p-value should be less than 0.01.
Beta Error:
Error occurring when an alternate hypothesis is accepted, when it is false. This is also called as Type II error, Consumer’s risk. To reduce this this ‘Power’ of the test need to be high. The stronger is the power of the test, it is more sensitive in identifying minute variations. This can be done by increasing the sample size of the test.